OCR in Finance: How OCR Accounting Enahnces Financial Data Processing?
OCR in finance streamlines data processing by converting paper documents into digital text. This boosts accuracy, speeds up workflows, and enhances efficiency for invoice handling and customer onboarding tasks.
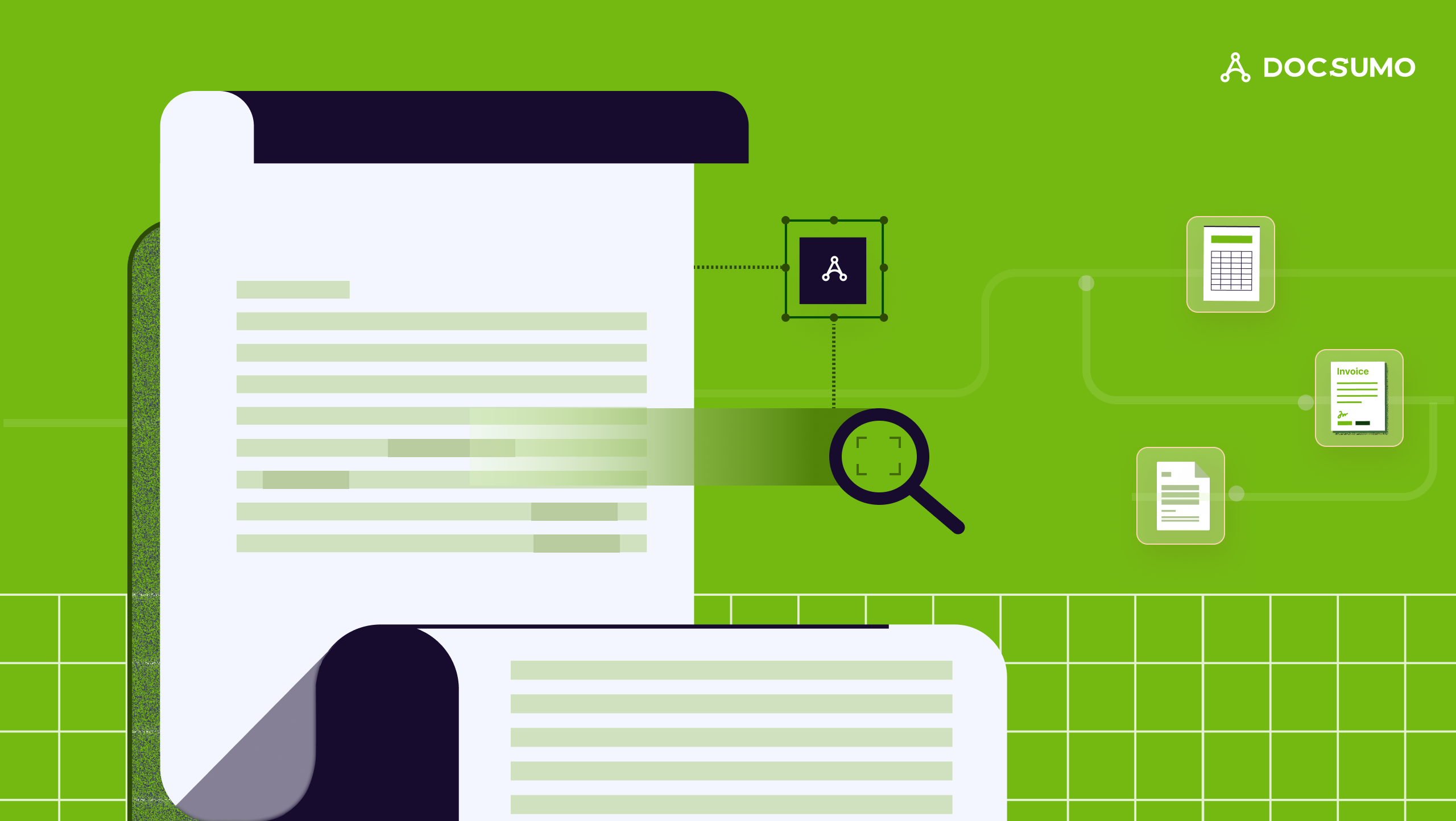
No matter what your organization deals with, one thing in common is dealing with loads of data. Be it financial statements, invoices, receipts, or others, manually keeping data entries from these papers can be a time-consuming and tiresome job.
According to McKinsey, a typical employee in the financial services sector spends 30% of their time each week on activities that can be automated using currently available technologies. OCR is here to the rescue.
By converting paper documents into digital data with a few mouse clicks, OCR streamlines workflows in the finance industry and makes them considerably more efficient, accurate, and productive. This blog will cover OCR in finance, its benefits, and more.
What is OCR, and how does it work for financial documents?
Optical character recognition (OCR) is the electronic translation of typed, handwritten, or printed images into machine-encoded text. OCR allows many paper-based documents in various languages and formats to be transformed into machine-readable text, simplifying storage and making previously inaccessible material available to anybody with a single click.
Optical character recognition (OCR) is the electronic translation of typed, handwritten, or printed text images into machine-encoded text. It allows many paper-based documents in various languages and formats to be transformed into machine-readable text, simplifying storage and making previously inaccessible material available to anybody with a single click.
The process of automating data extraction from financial documents like Invoices and form OCR works in three phases:
1. Pre-Processing
To start, the equipment part, which can be any kind of optical scanner, changes over the record's actual shape into a computerized picture. For instance, assuming there's a report on a piece of paper, the equipment part delivers an advanced duplicate of that identical archive.
During this cycle, the OCR technology must characterize the spaces of interest in the picture. For this situation, the spaces of interest are the ones that contain text, thinking about the unfilled areas as invalid.
That cycle is generally alluded to as changing the picture into foundations (white, clear regions) and characters (obviously dull areas).
2. Character Recognition
Once the backgrounds and characters have been separated, the process of determining the exact contents of the characters from scanned documents begins. The dark spots or characters identify numbers and letters. These features are studied in short parts rather than in bulk.
Typically, this refers to a single word at a time if the AI successfully understands the language and characters of the text and the content is plain to read.
Pattern recognition or feature extraction is the particular approach for character recognition, with the latter being used to recognize newer characters. This can be done in two sub-phases: Pattern Recognition and Feature Extraction.
3. Post-Processing
Later, each character in a given record is recognized. They are then converted to an ASCII code that can be stored for additional utilization. Sadly, no framework is idiot-proof, and it is not even the best one on the planet.
That is why most OCR frameworks complete a post-handling stage that twofolds the effort to examine the underlying result.
For instance, the characters 'O' and '0' can be almost indistinct, particularly when penmanship is involved. This makes the post-process stage significant for precision.
OCR and its Application in Business and Finance
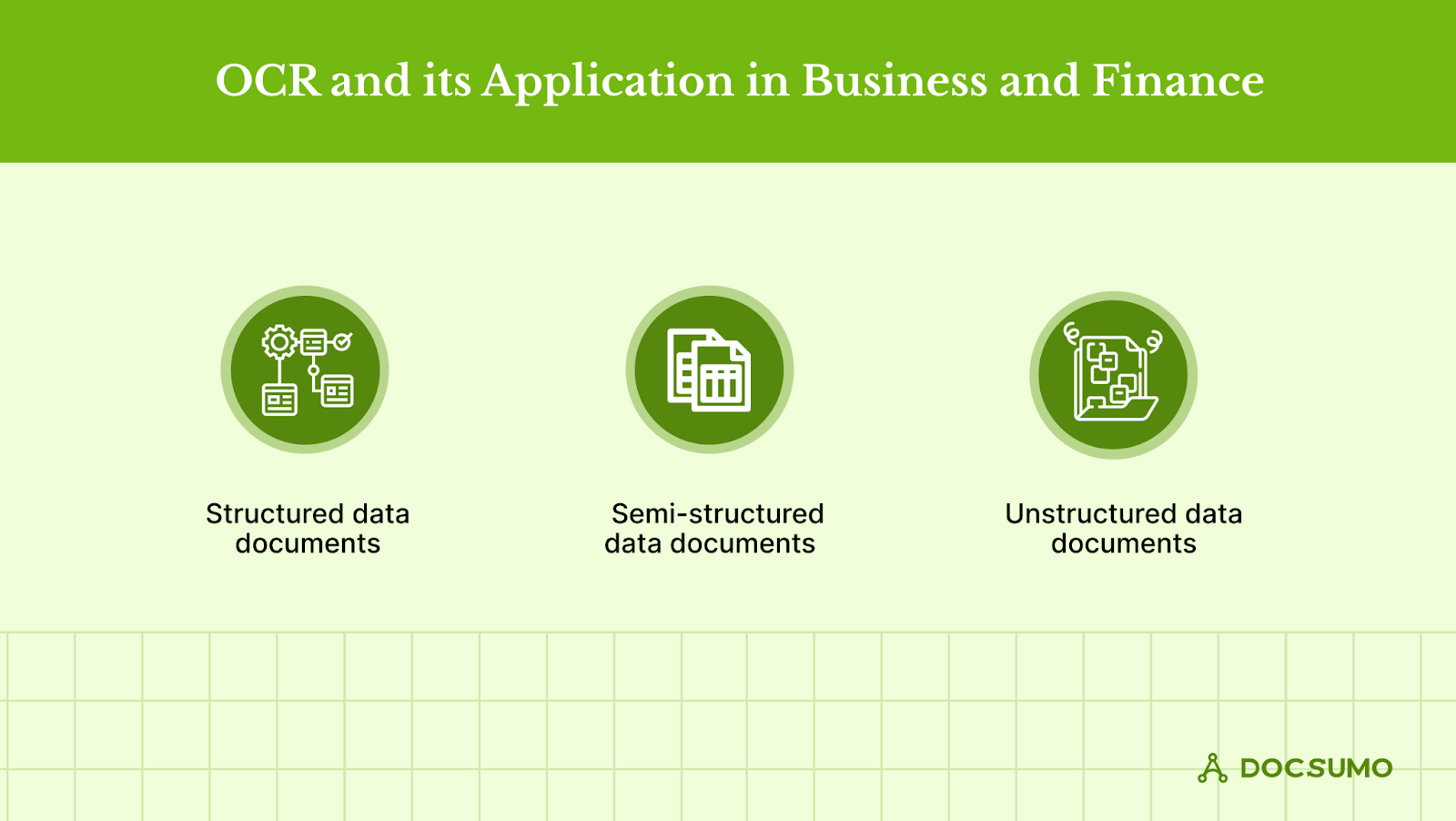
The absolute, generally prevailing, and normal utilization of OCR financial statements is document scanning, credit card scanning, data entry, and many more.
Financial documents can be classified into three types:
A) Structured data documents
B) Semi-structured data documents
C) Unstructured data documents.
1. Structured data document
A structured data document has aspects that can be addressed for successful analysis. It has been structured into a formatted repository, which is commonly referred to as a database.
Structured data refers to any data that may be stored in an SQL database in the form of a table with rows and columns. It includes relational keys and can be readily mapped into pre-designed fields.
How OCR works for structured data document processing
For most structured data documents, the algorithm is divided into three parts:-
- First is table detection and cell recognition using Open CV.
- Second is thorough cell allocation to the appropriate row and column.
- Third is extracting each allocated cell using Optical Character Recognition (OCR).
Clear and detectable lines are required for successful cell identification. Tables with broken lines, gaps, and holes result in worse recognition, and the algorithm frequently misses cells that are only partially encompassed by bylines.
Some papers may contain broken lines, which may interfere with data extraction. However, data processing techniques can also accomplish this.
2. Semi-structured data document
Semi-structured data has yet to be recorded or prepared in traditional ways. Because it lacks a set schema, semi-structured data does not adhere to a tabular data model or relational database format.
However, the data is somewhat raw or unstructured; it has certain structural features, such as tags and organizational information, that make it simpler to examine.
Examples of semi-structured documents include P&L statements, IRS Forms, Acord Forms, Bank statements, and Invoices.
How OCR works for semi-structured data documents
The position of key identifiers and checkboxes on semi-structured forms varies with the data fields. This is a difficulty for template-based OCR software since it may collect inaccurate data elsewhere on the page.
To discover a data point's 'position information,' data extraction from semi-structured forms employs business rules. These criteria are predicated on the notion that the extracted data is always in the same relative position to a defining feature.
3. Unstructured data documents
Unstructured data/documents are exactly as they sound – information that follows a freestyle design along these lines, with no set construction.
You would figure out that unstructured arrangements would be physically filtered. However, that is simply false. Unstructured information found in agreements, articles, letters, reminders, and more can be caught with the present progressed OCR Capture calculations.
How OCR works for unstructured data documents
The information extraction (IE) method extracts functionally organized information from unstructured data, such as entities, relations, objects, and events.
The information collected from unstructured data is utilized to prepare data for analysis. As a result, efficient and accurate unstructured data transformation in the IE process increases data analysis.
IE is conducted on unstructured data by combining several NLP-based techniques such as Named entity recognition (NER), Relation extraction (RE), Event extraction (EE), and salient facts extraction. The analysis can be carried out using these standard methods.
Benefits of OCR for Financial Documents
OCR has a crucial role to play in finance. Here are some of the benefits:
1. Enhanced Efficiency
Banks use OCR technology to speed up processing of customer documents needed for KYC. OCR technology boosts efficiency. It automates pulling data out of invoices and other critical financial documents. Employees no longer need to manually enter information from invoices, receipts, printed or scanned paper, and other sources.
Instead, that information is scanned and converted to a workable digital file in seconds. Employees save hours that otherwise would have been spent entering data. These efficiencies can mean invoices that took an hour to process now take minutes to approve and pay.
2. Improved Accuracy
One of OCR's most useful advantages is its inherent ability to eliminate human error virtually. When humans type in data, they are susceptible to typos, poor translation, or lack of thoroughness.
On the other hand, OCR is systematic and precise in its extraction and transcription of data—keeping financial records exact and trustworthy for those who need to make decisions based on them.
For instance, when processing documents for account opening, the data are taken automatically, so there are fewer chances of errors.
3. Cost Savings
Implementing OCR financial statements can also help companies save costs. Automatic data extraction requires less manual labor to do the desired work, reducing operational costs. That is especially true for financial departments that have to deal with many documents.
Less human intervention will result in fewer mistakes, thus reducing reworking and administrative expenses. These savings will add up in the long run, creating a leaner, cost-efficient organization.
4. Better Compliance
Financial regulations and compliance requirements are stringent. Maintaining accurate records is crucial for meeting these standards. OCR helps ensure that financial statements and documents are accurately recorded. They are easily retrievable, simplifying compliance with regulatory requirements.
With digitized records, conducting audits, tracking document histories, and ensuring all necessary documentation is in place becomes easier. It further reduces the risk of non-compliance and associated penalties.
OCR in banking helps to detect any discrepancies in financial documents during audits and expense reporting, thus leading to fraud detection.
5. Increased Accessibility
With OCR, financial documents are converted into digital formats that can be accessed from anywhere, at any time. This increased accessibility facilitates better collaboration among team members, especially in remote or hybrid work environments.
Digitized documents can be quickly retrieved, shared, and reviewed, improving overall workflow and ensuring that critical information is readily available when needed. This flexibility enhances productivity and supports more efficient decision-making processes.
Use cases of OCR in the Finance Industry
OCR technology is revolutionizing the finance industry. It enhances operational processes and efficiency across various functions.

Here’s a closer look at how OCR is making a significant impact:
1. Customer Onboarding
A report suggests that organizations can lose 74% of potential customers due to a complicated onboarding process. Imagine entering a bank or financial institution and waiting ages to open a new account. OCR financial statements is changing that narrative by speeding up the customer onboarding process.
OCR speeds up and improves the process by scanning and extracting data from identity documents like passports and driver’s licenses. This means less waiting time for customers and a smoother overall experience.
For financial institutions, it enhances customer satisfaction and helps meet KYC (Know Your Customer) regulations more effectively. With accurate data entry from the get-go, the onboarding process becomes much more streamlined and less prone to errors.
2. Invoice Processing
Handling invoices can be tedious, especially when it involves a mountain of paperwork. A report claims that handling invoices manually could take up to 14.6 days.
OCR simplifies automated invoice processing by automating the extraction of critical data from these documents. Instead of manually entering details like amounts, dates, and vendor information, OCR technology quickly pulls and digitizes this data.
This automation drastically reduces processing times and minimizes the risk of human error. As a result, financial teams can manage a larger volume of invoices without getting bogged down, leading to faster approvals and payments. The efficiency gains are significant, freeing up time for more strategic tasks.
3. Bank Statement Analysis
Bank statements are crucial for understanding financial health, but analyzing them can be cumbersome when dealing with piles of paper. OCR in banking transforms these paper statements into easily accessible digital formats.
This conversion allows financial analysts to quickly pull up, compare, and analyze data from different accounts and periods. With everything digitized, spotting trends and anomalies becomes much more accessible, helping analysts make more informed decisions.
Whether for budgeting, forecasting, or financial reporting, OCR provides a more precise and more organized view of financial data.
4. Loan Approval and Credit Card Processing
Approving loans or credit cards often involves a lot of paperwork and verification. OCR speeds up this process by automating the capture and processing of required financial documents. OCR handles it quickly and accurately instead of manually reviewing and entering application data.
This speeds up approval times and enhances the accuracy of data used for decision-making. Customers benefit from faster approvals, while financial institutions gain more reliable creditworthiness assessments, leading to better decision-making and improved customer satisfaction.
5. Fraud Prevention
Fraud is a serious concern in the financial industry, and OCR is critical in preventing it. By ensuring that the data used in transactions is accurate and unaltered, OCR helps identify discrepancies and potential fraud in financial documents.
The technology can spot anomalies in text or figures, which might indicate fraudulent activity.
This early detection is crucial for protecting both financial institutions and their customers. By flagging potential issues before they escalate, OCR helps maintain the integrity of financial transactions and safeguards against fraud.
Are OCR-Based Systems Completely Reliable? If Not, What Are Their Limitations?
Apart from having advantages, OCR also has some major limitations like:
- OCR text works efficiently with printed text only and not with handwritten text, so it might lead to inaccuracy which is not desirable in finance.
- OCR solutions are highly efficient for good quality
- of data but if they are fed improper data, then this might lead to inefficiency.
- All the documents must be checked carefully after processing and should be manually corrected if differences are found.
- OCR systems are not 100% accurate, and mistakes are likely to be made during data extraction and processing.
Choose the Best OCR Engine for Your Documentation Purposes
Choosing the right OCR engine is crucial for efficiently converting paper documents into digital formats. A high-quality OCR engine can improve data accuracy, reduce processing times, and streamline document management.
When evaluating OCR solutions, it's essential to consider how well they handle various document types and formats and their ability to integrate seamlessly with your existing systems.
The right OCR engine should digitize your documents accurately and enhance productivity by automating data extraction and reducing manual entry tasks.
For businesses in the finance sector, where precision and speed are critical, selecting an OCR engine that meets these needs is particularly important. The solution should be able to manage large volumes of documents quickly while ensuring data integrity.
- Docsumo delivers precise text recognition, ensuring data is captured with minimal errors. This accuracy is crucial for maintaining the integrity of financial information.
- The platform quickly processes large documents, enabling rapid data extraction and analysis. This efficiency helps speed up your financial operations.
- Docsumo integrates smoothly with your existing systems and software, making it easy to incorporate into your current workflow without causing disruptions.
- Docsumo ensures that your data remains protected, adhering to high security and compliance standards to safeguard sensitive financial information.
Explore how Docsumo can enhance your finance workflows and improve efficiency today!
Frequently Asked Questions
What is OCR in banking terms?
OCR in banking automates the extraction of data from financial documents like checks, invoices, and bank statements. This technology helps speed up processes and reduce manual data entry by converting printed or handwritten text into machine-readable data.
What is OCR full form?
The full form of OCR is Optical Character Recognition. It refers to technology that converts different types of documents—such as scanned paper documents, PDF files, or images—into editable and searchable data.
What is the full form of OCR billing?
The full form of OCR in billing is Optical Character Recognition. In this context, OCR is used to digitize and process billing statements and invoices, making it easier to manage and analyze financial data.
What is the full form of OCR in banking?
The full form of OCR in banking is Optical Character Recognition. It automates data extraction from various banking documents, such as checks and account statements, to streamline operations and improve efficiency.